2. June 2022 By Lilian Do Khac
Trustworthy AI – the holistic framework and what parts of it will be tested in future
The trustworthy characteristics of an artificial intelligence (AI) are addressed in the context of Trustworthy AI. The spectrum of requirements for Trustworthy AI ranges from the AI’s design to its functions, all the way to its operation. These trustworthy characteristics are an essential part of the overall organisational trust framework when it comes to making human-AI collaboration successful and sustainable. Sustainable human-AI collaboration means more than just meeting regulatory requirements. Standards that make it possible to quantify and compare data are currently being developed for this purpose. Trustworthy AI covers an interdisciplinary range of topics, and the implications affect almost every industry. This complexity arises from the nature of the technology, direct interaction with the environment and the current fast-moving nature of normative requirements. At this point, you’re justifiably asking yourself: where should we start, and where’s the end? A holistic framework is used to create an overview, which, in turn, can be broken down into small parts. It’s easier to start with the individual parts and then work on managing Trustworthy AI as a whole. In the following, I’d like to briefly present such a holistic framework and highlight individual aspects of it. I’m deliberately only looking at business processes in this blog post due to the fact that Trustworthy AI for products is subject to several other requirements and that managing it most likely doesn’t necessitate an entire management body. Specific individual aspects will be explored in more depth in follow-up blogs. Furthermore, I’ll compare the holistic framework with the VDE SPEC 90012 V1.0.
Why is AI a ‘management problem’?
A definition of AI from a management perspective has finally been introduced in the field of management research. Yea, it’s the hundredth definition of AI, but this one’s really cool! In this definition, AI is the level of technology that’s beyond the edge – at the bleeding edge – one step after the cutting edge. So if cutting-edge is the edge of the technological status quo, then bleeding-edge is what we can’t do yet. Whenever we can do something new, this edge extends further. It almost sounds like Hansel and Gretel, only without an end. However, there’s a lot more to it than that. It means that we won’t always maintain a learned state of AI (equilibrium) that’s possibly merged into intelligent automation, especially since the world will most likely continue to turn, and the data situation along with it. So we have to constantly recalibrate. This oscillating phenomenon in human-AI collaboration means that the division of labour is continuously changing. This is also accompanied by the fact that responsibilities may be oscillating. So, if you introduce more and more AI into existing workflows as part of a human-AI collaboration in order to leverage more potential, you’ll inevitably also have to deal with the oscillation phenomenon just described.
The AI affects the human, the human affects the AI; et voilà, the ‘software tool’ becomes a new kind of team member in the organisation. This implies an urgent need for proper integration into the work structures and ensuring proper work processes and the expected quality of the result types. For 50 years, the development of AI has mainly been driven from a technological perspective. The sociotechnical view has been lagging somewhat behind. Integration doesn’t just mean integrating into an existing IT system. The integration required here also has a significant organisational component. The human-AI system must abide by the market rules. Trust plays a significant role in a social context. In the field of organisational research, it’s said that trust plays a mediating role for cooperation.
Trust – sounds kind of romantic, but what is trust exactly?
Trust is a huge topic in organisational theory. Anyone who has studied business administration will certainly remember the principal-agent theory, where information asymmetry prevails between the client (principal) and the contractor (agent). This can be sufficiently resolved in various ways, for example, via an intermediary medium, such as a contract. The purpose of the contract is to create trust so that both parties can work together. From an organisational perspective, hierarchical and management structures have developed with the purpose of promoting (efficient) cooperation throughout the entire organisation. Concluding contracts and defining rights and obligations doesn’t work so well between an AI and a human. The AI has no intrinsic motivation to adhere to normative conditions unless the norm was explicitly programmed into the AI (co-workers who believe in hard AI will probably disagree with this – I personally am on the ‘soft AI’ team). To do these things, there must first be a consensus among us humans about everything labelled as ‘normative’. There are now hundreds of different opinions on AI ethics guidelines alone.
There’s no clear-cut definition of trust, which means that the trust construct and AI have quite a lot of similarities. Psychosomatic factors are difficult to measure (for the time being, labour law wouldn’t permit measuring these factors – for example, with electroencephalography [EEG], in other words, neurological values). Tailoring AI to factors that are difficult to measure is therefore even more difficult. So is it even possible to trust an AI, or is that not just a thing between people (and animals)? Researchers are not in agreement. People – as opposed to machines – mirror social behaviour patterns. And the expectation of a machine’s ‘performance’ is higher than what’s expected of a fellow human (back in college, ‘the machine’ was lauded as not sweating, not sleeping and not tired). People are allowed to make errors, whereas a machine is met with a very low tolerance for errors. This is also the case when it comes to gaining trust. While people have the ability to win more trust from fellow humans, machines gradually lose peoples’ trust when errors recur. The most well-known concept of trust in the organisational context comes from R.C. Mayer and is defined as follows: ‘Trust is the willingness of a party to be vulnerable to the actions of another party based on the expectation that the other will perform a particular action important to the trustor, irrespective of the ability to monitor or control that other party.’ To this end, the researchers formalise three trustworthiness factors: 1) ability = the capacity to complete tasks or solve problems, 2) benevolence = doing no harm and 3) integrity = abiding by the rules. These factors are mediated by one’s own propensity to trust AI and the perceived risk situation.
Trustworthy AI – design elements
Can these trustworthiness factors be broken down in more detail? Yes, they can. The diagram below shows my understanding and synthesis of Trustworthy AI based on research and experience. The requirements of Trustworthy AI are therefore met by technical functions and via the governance of the organisation (inner area of the diagram). Technical functions include reliability, robustness and explainability (blue area in the diagram). Organisational functions include things such as defining adaptive interaction margins, metrics and training (yellow area in the diagram). An organisation interacts with its environment – in this case, we’re interested in its interaction with the market. Market rules are the result of regulation stemming from legal requirements or industry standards. ESG requirements also play an increasingly important role in the acceptance of AI applications. Certifications carried out by independent third parties foster trust in the AI application. In the following, we’ll attempt to take a selection of the key points one level deeper.
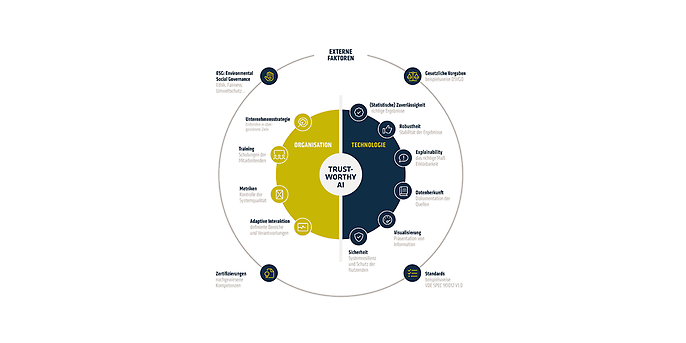
adesso Trustworthy AI Framework
The technical dimension
Most of the technical requirements for a trustworthy AI application have something to do with the ‘ability’ factor. These requirements include sub-factors, such as reliability, which can be achieved by implementing best-practice software engineering methods and complying with statistics-based metrics. Resilience is an essential sub-factor as well. The system must be able to properly function or react in the face of environmental changes and the changes in data they cause. One of the things you hear mentioned in technical jargon is ‘drift’. And there are detection and migration methods for dealing with it. A ‘drift’ is a change in data – especially with regard to the target variable(s) – that consequently affects the model. And we mustn’t forget about explainability. Explainability is probably one of the most discussed subcategories – and not without reason – as it’s connected to virtually every other subcategory (see how VDE SPEC 90012V.1 T3 – Intelligibility suggests conducting an evaluation). Unfortunately, explainability is haplessly vague when it comes to exchanging communications and when it comes to your planned intention. Thus, transparency gets mixed up with interpretability or interpretability with explainability and other synonyms. The actual objective, namely explainability with respect to the target audience (books and films have a target audience as well, after all), is forgotten. We make a rough distinction between two levels of explainability according to the target audience, one of which is the transparency that the system is supposed to portray without retouching anything, thereby making it explainable. Transparency is suitable for developers, data scientists and, if necessary, auditors who have a data science background and whose objective it is to conduct a comprehensive audit of the system or develop it further. In order to do this, there must be complete transparency that’s true to the use case. An excerpt detailing the transparency is probably absolute overkill for the layman. An end-user may just want to understand why a particular decision (usually the unfavourable one) was made and have a fair opportunity to challenge it (for example, in a bank lending situation). This corresponds less to a need for transparency and much more to a need for interpretability. This includes clear and bite-sized communication. Methods such as using counterfactuals (OII | AI modelling tool developed by Oxford academics incorporated into Amazon anti-bias software) or presenting ‘what-if’ scenarios (what if by ‘trying out’ different input data...) (see What-If Tool (pair-code.github.io)) come quite close to what’s needed. The smorgasbord of methods used in Explainable AI is huge and would probably go beyond the scope of this article. There will be another blog post to address this. Anthropomorphic characteristics refer to the way AI applications represent communication. One of the things that has an influence on trustworthiness is the ‘avatar’ in which the AI ‘finds itself’ (keyword: ‘uncanny valley theory’ – a paradox which maintains that the more realistically ‘figures’ are portrayed, the less ‘trust’ people place in them. Roughly speaking, a distinction is made between three main forms: the robot, the virtual agent (for example, the Microsoft paperclip Clippy) or the embedded agent (rather, invisible agent, such as recommender systems in Netflix). A distinction can also be made according to gender (ever wondered why voice assistants are given women’s voices by default?)). In certain application scenarios, human-like attributes are desirable and promote trust, and in others, not so much. So then, how can such attributes be specifically built into the AI? For example, etiquette can be engrained in the communication output. If you’d like to learn more on the topic of privacy, check out the adesso blog entry ‘Trustworthy analytics’ by Dr Christof Rieck and Manuel Trykar.
The dimension beyond the company
There are also external market factors that influence the organisation and impose framework conditions on the AI application. If these market rules are fulfilled (for example, in future the requirements of the EU Artificial Intelligence Act), then the ‘integrity’ factor in the trust model described above is achieved as well. A clear subcategory is the ESG requirements, which involve complex issues such as ethics, fairness and environmental protection. While environmental protection is complex yet still measurable and therefore manageable (see how VDE SPEC 90012V.1 F3 – Ecologically Sustainable Development suggests conducting an evaluation), this is not so easily the case with ethics and fairness. Ethics is a tricky concept on which there is also no general consensus – in other words, ethics is alive and continuing to evolve. It’s possible to take a data-based approach to this topic and address the classification configuration or formulate control-related proxy variables and use data provenance methods (documenting the data’s origin, see how VDE SPEC 90012V.1 T1 – Documentation of data sets suggests conducting the evaluation) as part of a documentation-based approach – there are already a handful of tools for doing this. It’s also possible to take a normative approach to this topic and consider the effect of the decision for the individual data subject (counterfactuals). Now the question is: if ethics is somehow continuing to evolve – and recently it’s been evolving quite rapidly – and we don’t agree on some of the issues (if not many of the issues), then ethics can’t even be ‘programmed’ into AI, can they? That somehow rings true. Managing ethics issues concerning the AI application must hold some kind of governance function within the company. Instituting a ‘charter’ is not enough. It’s also not enough to simply appoint a ‘Chief of Ethics’ and use them as a scapegoat for every ethics-related question. We have already seen this in the past. If you want to get serious about this issue, you have to establish a serious governance function. That sounds like a lot of work at first and implies a certain time frame for setting up such functions. Does this mean that AI ethics would begin to be addressed later on? Certainly not. There are what are called ‘kindergarten ethics’ that can begin to be addressed without any problems. Everyone knows – and hopefully this won’t present any cultural differences – that a car shall not kill another car.
The dimension within the company
A handful of measures for the organisation are now emerging. These measures must cover the organisational structures of management strategies regarding the AI application in addition to the Trustworthy AI by design aspect of the technical realisation process. For many risks, the adaptive calibration approach can be used. The assumption is that over time, as well as in more extreme events, the system will no longer behave as perfectly as it should and humans should intervene – with the human-in-the-loop model, yet somehow adaptively implemented, so as not to switch from considerably automated to completely manual. After all, not everyone can jump right into a cold shower. What are called ‘level of automation scales’ in the field of science are available for this purpose and serve as a source of inspiration. Now, when does the human know when to intervene? In order for them to know, the AI must somehow make itself ‘noticeable’. To do this, the AI must use an alarm function. Everyone knows how it is: the more often the alarm beeps in a startling way, the more likely it is that it will no longer be taken very seriously or that you don’t feel like dealing with it at all because the threshold was set too sensitively. In the worst case, the person even loses confidence in the application. It’s a bit like the boy who cried wolf. Therefore, when conducting research and in some practical applications (for example, the weather report), the approach taken is to map out a probability of occurrence (‘likelihood alarm systems’ [LAS]). This approach promises a higher degree of acceptance and a reduced risk of human error when working with AI. So what happens when humans really do have to intervene? This is conceivably a bad thing after a long phase of high levels of automation. It’s similar to when you haven’t done any sport for years and then suddenly have to sprint after the last train in the middle of nowhere, and your phone doesn’t have any reception. The scientific term for this is ‘human-out-of-the-loop’. Therefore, it’s necessary to continue training users in order to counteract overconfidence and keep them fit (by simulating different types of malfunctions as part of the training, for example) or also to increase their awareness of their own performance in conjunction with the AI. We humans are also notorious for our tendency to overestimate ourselves.
How can I ‘measure’ my AI trustworthiness?
Now that was quite a lot for an initial rough overview, what with all the aspects to consider. On the technical and organisational level, the question is whether this can be made comparable. The VDE SPEC 90012 V1.0 presents an approach to conducting quantified evaluations. The VDE SPEC system is based on the VCIO model and stands for Value, Criteria, Indicator and Observables. The values are consolidated from the criteria that can be evaluated on a scale from A (highest) to G (lowest) based on the indicators gathered from the observations. The values approach pretty much perfectly coincides with the psychosomatic trust construct. Therefore, it comes as no surprise at all that almost every aspect in the adesso Trustworthy AI Framework would be tested. The minimum requirements per value differ depending on which industrial domain (and perhaps also on which specific application) the system is applied to. Such ‘benchmarks’ can be derived from risk domains, as shown in the four examples below. Heads up! It’s very illustrative. There’s likely to be a high bar for privacy and fairness values in an application where the level of ethical risk is relatively high (in the medical field, for example). In an application with a high level of time-loss risk, privacy and fairness will play a rather subordinate role and, in contrast, reliability will become much more important. The five values from the VDE SPEC are mapped onto adesso’s Trustworthy AI framework in the graphic below. Which aspects does the VDE SPEC not explicitly check at the moment? Anything that wouldn’t break rules if it wasn’t done. This would include useful management strategies, training employees and anthropomorphisms. Those who would opt not to establish useful management strategies for human-AI collaborations are likely to exit the market soon; so it’s in their own interest to do so. Those who don’t establish good training programmes for their employees and build anthropomorphic characteristics into AI systems have a completely different problem. Let’s assume that each company manages to live up to their industry-specific values to a minimal degree. Then the only factor that’s still more important than surpassing this minimum degree is the differentiation factor. This is the path to increasing effectiveness and efficiency. The characteristics that AI doesn’t possess yet are the basis for maximising potential. These include human qualities, such as emotionality and creativity. People in the scientific community are already talking about ‘feeling economy’. The mass customisation movements have shown that end customers are demanding more individual services and products. These are the solution spaces that are so different that only a human being would be able to deal with them.
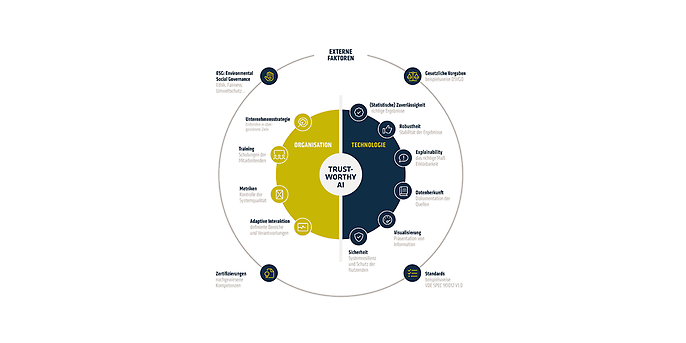
Gegenüberstellung adesso TrAI Framework zu VDE SPEC 90012 V1.0 Values
Our brief excursion into the various risks and their effects on AI requirements already gives an idea of the upcoming state of affairs regarding risk management functions. So what can you take away from all of this? It’s a killer topic. Doing nothing will turn out to be fatal, because there is a lot to be done and the decision lies somewhere between losing the connection to the AI transformation or not being able to manage the AI transformation.
You will find more exciting topics from the adesso world in our latest blog posts.