12. July 2022 By Lilian Do Khac
Trustworthy AI – a wild tension between human and machine interaction
Artificial intelligence (AI) continues to evolve and the limits of the status quo of feasibility are slowly but surely being reached. The question of how the company plans to deal with these challenges is coming up in more and more management meetings. Over the past 50 years, human-machine collaboration has been driven more from a technical perspective than on the basis of formalised social requirements. There are various names for this specific challenge, such as ‘socio-technical system’, ‘human-machine interaction or collaboration’, ‘trust AI’ and ‘trustworthy AI’. The core thing that all of these share is the desire to integrate AI into existing processes in a way that both makes economic sense and that is socially and environmentally acceptable.
Dr Alondra Nelson, Head of the White House Office of Science and Technology Policy (OSTP), put it in a nutshell during the kick-off meeting of the US National Artificial Intelligence Advisory Committee (NAIAC) on 4 March 2020: ‘[...] we need to ensure that we harness the good of AI and banish the bad [...]’. After taking a bird’s eye view of the holistic framework of trustworthy AI in the first blog post of this series, I would now like to delve deeper into the topic. In this blog post, I will take a look at the core requirement in the trustworthy AI field, namely ‘trust’. I will also elaborate on aspects regarding the division of labour between humans and machines.
How everything is moving from the machine-driven industrial revolution more and more into the cognitive-driven social revolution
In order to understand what the challenges at the heart of human-machine interaction are today, you first need to understand where they come from, or to put it another way, understand what the legacy behind them actually is. Let’s take a brief look at the various different industrial revolutions. By the end of the 19th century, mechanisation had enabled the beginning of the first industrial revolution (Industry 1.0), which saw smaller farms be replaced with larger factories, and small grocer’s shops with standardised businesses. The second industrial revolution (Industry 2.0) followed on from this and further enabled the mass production of goods. This period also saw questions starting to be asked about how large groups of people should be organised along the workflow with machines. The first approaches to classical management theory, which still resonate today, were developed as part of this, with Frederick Taylor, Henri Fayol and Max Weber being prominent exponents. Automation saw these approaches developed further in the third industrial revolution (Industry 3.0). However, an essential developmental feature is reflected here when considering the social aspect in the organisational structure – the human being became more of an operator for the machines and the one who answered questions regarding monitoring instruments. Sociological and psychological aspects – essential aspects of neoclassical management theory – came more to the fore. The Hawthorne studies, which investigated how the design of a person’s immediate working environment had an impact on their productivity, were a prime example of this.
As part of this, the term ‘automation’ was coined for common use. Not even a half a century later, we have arrived where we are now – namely in the fourth industrial revolution (Industry 4.0), otherwise known as ‘the digital age’, and the increased development of modern management theories. Modern management theories are about what non-classical management theory is – the work is much more analytical and data-driven. This is because people can now access quantitative properties, as well as focus more on complex interrelationships in detail with system-theoretical approaches, which correspond to the prevailing approach. Whereas in the previous industrial revolutions it was predominantly the individual that was absorbed into the greater whole, now the individual is once again coming to the fore and becoming the centre of demand – in other words, it is becoming more people-centred. Rather, the technical fabric merges into the social fabric, leading to human-machine collaboration – but watch out, collaboration is not cooperation. The aspect of ‘trust’ is the cognitive bridge that needs to be built to make this type of collaboration a success, and this will be the challenge of the coming decades. Some scholars, mainly from the research branches around decision theory – including operations research – refer to this development as, among other things, Industry 5.0.
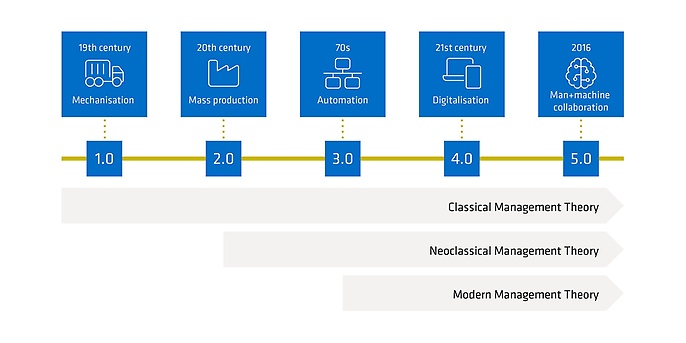
Trust – a romantic appeal from two perspectives
The use of AI applications has significant implications on the management dimension and, according to Sebastian Raisch and Sebastian Krakowski,
should be understood more as ‘[...] a new class of actors in the organisation [...]’. According to the dictionary, a tool is defined as ‘an object shaped for specific purposes, with the help of which something is [manually] processed or manufactured’. In turn, the dictionary defines the term actor as ‘a participant in an action or process; a person who acts’. An actor is therefore something that can improve itself through learning.
In the mid-1990s, two main formal trust models emerged from two different research directions. One stems from research in the social sciences with the aim of explaining a better understanding of interpersonal cooperation in complex organisational structures. The other stems from research in automation science with the aim of better understanding the human automation interaction and thus highlighting specific characteristics of the technology used. Social science research looks at actors and sees trust as moderated by interpersonal characteristics (AI competencies, AI integrity and AI benevolence). Automation science, on the other hand, focuses on the characteristics of automation (the reason for AI, the process in which AI is embedded and AI performance).
Inside the collaboration paradox
In my last blog post on trustworthy AI, I briefly described the management problem that AI causes. The crux of the matter was that AI capabilities are constantly evolving, and thus human-AI collaboration is subject to constant recalibration. In their impressive article ‘Artificial Intelligence and Management: The Automation-Augmentation Paradox’, Sebastian Raisch and Sebastian Krakowski describe how the use of AI applications in companies is closely intertwined with time and space, creating a paradox. Augmentation in this case describes human-AI collaboration, while automation describes the complete assumption of human activities. This is not meant to be an excursion into the Sci-Fi world of Interstellar, but rather to highlight what is important in modern management practice.
It is not one or the other – augmentation or automation – that management should emphasise. Overly focussing on automation would almost be an act of regression from an industrial revolution point of view. If there is too much augmentation, the negative side effects of AI that people fear will probably occur too often.
The contention just described is admittedly already on an intergalactic level. Approaches to transfer the paradox to an operational and tactical level, which can be simultaneously defined as requirements and subsequently implemented, are referred to as ‘adaptive automation’. There are different scales for this – starting from purely manual task processing (which you can see in the figure below on the left under ‘manual execution’) up to fully automated task processing (which you can see on the right under ‘automation’). These two static expressions are fixed and accepted across the board. The ‘hybrid system’ is where the line becomes seriously blurred. A hybrid system can mean, for example, that an AI pre-selects the decision and executes it after human approval. This would be what’s known as an assistance system. A somewhat more independent hybrid system would be, for example, a system that directly implements a pre-selected decision and informs the human about it. This would see humans still have a ‘veto’ and they could actively intervene in the ‘doing’. However, the purpose of the respective static interaction level is not to dwell on it, but to create the possibility for people to calibrate themselves within the right interaction level at the right time. Depending on the industry and, if applicable, the specific task with its specific requirements, there are different ranges of the possible interaction continuum. For example, full automation may never happen in some sectors or for specific tasks simply because of the ethical risks (see ‘ethical risk’ in the figure below). An appropriate level of augmentation, meanwhile, would maximise potential. You must also remember that there are use cases in which the risk of time loss can only be negated with full automation because by the time humans were able to intervene it would already be too late (see ‘risk of time loss’ in the figure below).
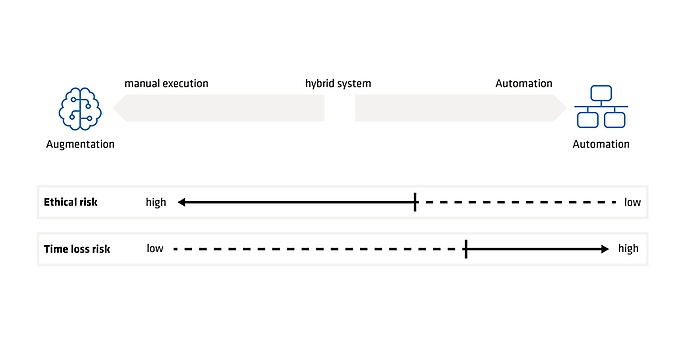
The current industrial revolution(s) will be clearly focused on hybrid systems and the interaction of social aspects as well as technical aspects for the next few decades. Trust is the link between these worlds and the focus of future AI implementation activities in organisations. The collaboration paradox will shape questions regarding modern management paradigms and raise the need for better calibration mechanisms between humans and machines.
You will find more exciting topics from the adesso world in our latest blog posts.
Also interesting: